08-08-2024
The Role of AI Technology in Revolutionizing Genetic Research
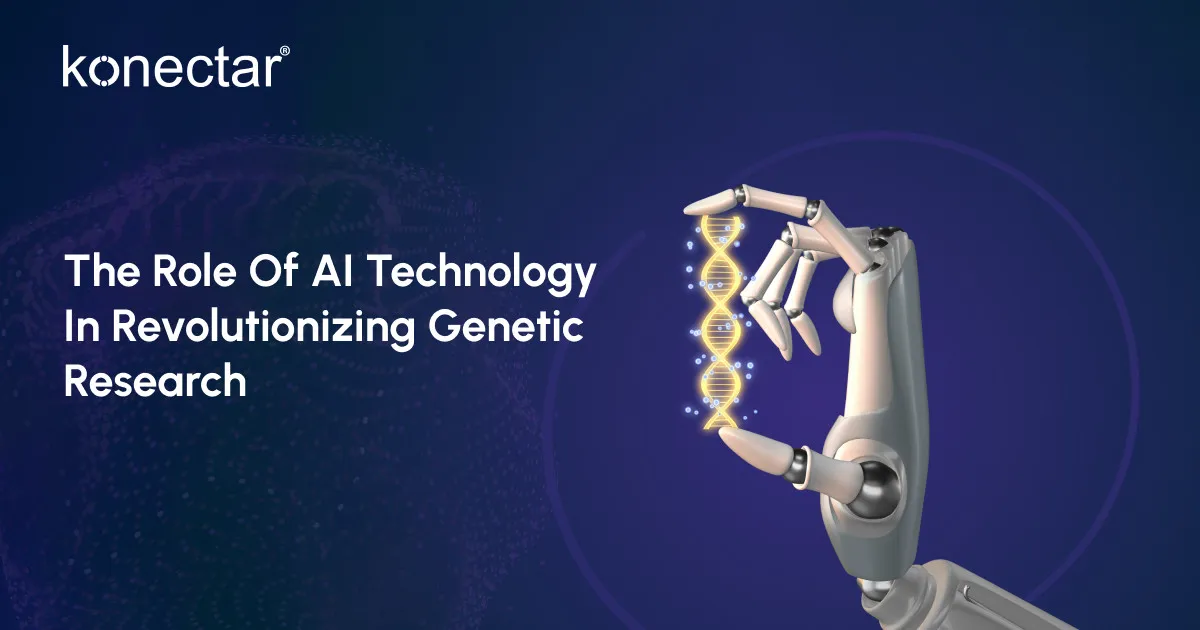
Genetic research has emerged as one of the most rapidly advancing fields globally. Driven by technological advancements, this field is rapidly uncovering the genetic basis of diseases. This has led to substantial progress in disease risk assessment, diagnosis, and treatment. Genetic engineering has seen remarkable strides over the past few decades, and the emergence and application of AI has further boosted the ability of healthcare providers to understand and manipulate genetic information for therapeutic purposes.
In this article:
Traditionally, drug discovery has always been a lengthy process that includes target identification, molecular simulations, and candidate prioritization. While the actual steps involved in drug discovery remain the same, AI has streamlined these processes and reduced the time taken to execute each step with remarkable precision and efficiency.
AI algorithms' ability to sift through vast datasets consisting of omics data, disease associations, and clinical trial outcomes has helped identify potential drug targets with utmost ease. Hence, AI is proving to be a game-changer in revolutionizing genetic research.
How is AI Transforming drug discovery?
Various ML algorithms are extensively used to process and analyze large volumes of data in tasks including clinical imaging, bioactivity predictions and virtual screening. One of the algorithms employed helps predict toxicity and the patient's response to the specific treatment.
Some models are for molecular target identification and clustering can help identify patterns with the data. Moreover, certain advanced algorithms are used to predict pharmacokinetic properties.
Computational models built on AI and ML are extensively used for chemical compound identification, peptide synthesis, drug monitoring, drug efficacy and effectiveness assessment, bioactive agent prediction, protein-protein interaction, protein folding, and misfolding identification. So you see, these AI algorithms have made the identification of lead compounds and toxicity challenges from chemical libraries, which comprise 106 million compounds, so much faster and easier.
Certain ML-based tools also help create the 3D structure of a target protein, which is an important step in the drug discovery process.
AI in Clinical Trials and Antibody Development
Antibodies form an integral and important part of the body's defense mechanism. They help destroy and get rid of foreign elements, including bacteria, fungi, toxins, and viruses from the body. Their ability to identify and eliminate these foreign organisms and molecules makes them important for preventing and treating various kinds of diseases. Creating therapeutic antibodies is a laborious process; it involves selecting the right candidate from billions of antibody sequences, and in many cases, it results in the identification of ineffective antibodies.
AI-based methodologies are proving to be a game-changer in antibody development, especially in small-molecule drug discovery. Due to recent developments in AI-based models for antibody development, many biotechnology companies are devising their own tools to assist in clinical trials.
Researchers at the University of Michigan have also developed new ML algorithms that can identify problem areas in antibodies. This will make them less likely to bind to non-target molecules, which is deemed a breakthrough, allowing scientists to modify specific antibody positions.
Implications and Future Outlook
Nearly 25 years after the first human genome was sequenced, whole genome sequencing has seen significant advancements. This process involves determining the sequence of the 6.4 billion DNA building blocks that make up an individual's genetic code.
Thus, the integration of AI into genetic research has accelerated innovation and generated hope for developing treatments for diseases and conditions that don't have any cure presently. Moreover, it has done great strides in personalized medicine and treatment accessibility.
The automation of complex tasks which would take months if not years otherwise has enabled life sciences companies to enhance decision-making. This all has reduced the time taken to discover drugs, making life-saving therapies more accessible and affordable globally.
Shedding light on the prospect of AI beyond scientific circles, it has generated interest of investors and stakeholders worldwide. Genetic research companies leveraging AI in drug discovery have attracted significant investments. This reflects the confidence in AI's transformative potential.
However, as AI continues to redefine genetic engineering and medicine, ethical considerations loom large. Life sciences and health-tech companies engaged in developing AI tools for genetic research have to deal with problems including data privacy, algorithmic bias, and AI's usage in decision-making processes.
Hence, safeguarding patient rights and ensuring transparency in AI-driven research is imperative. This will promote trust and mitigate potential risks associated with rapid technological advancements.
In conclusion, the convergence of AI and genetic research represents a watershed moment in scientific discovery. As we navigate this transformative era, collaboration between scientists, policymakers, and industry stakeholders will be crucial in harnessing AI's full potential while addressing ethical, legal, and societal implications. The future of genetic research is undoubtedly intertwined with AI, promising a future where innovative therapies are not just imagined but realized, offering hope to millions worldwide.
Life sciences companies looking to engage clinical trial investigators and key opinion leaders (KOLs) in genetic research can benefit significantly from konectar, an AI-powered HCP management platform. This innovative tool has been adopted by numerous biotech and pharmaceutical companies globally to enhance their research collaborations and streamline communications. To understand how konectar can support your initiatives, request a demo today!
FAQs
- How has AI transformed the drug discovery process in genetic research?
AI has streamlined drug discovery by analyzing vast datasets to identify potential targets, simulating drug interactions, and designing novel molecules. This has reduced the need for extensive genetic engineering laboratory testing and expedited the development process, which would otherwise take years. AI has made the drug discovery process quicker and more efficient.
- What are some significant technological milestones in genetic research?
Key milestones include the discovery of DNA's double helix structure in the 1940s by Watson, Crick, Wilkins, and Franklin laid the groundwork for modern genetics. In 1977, Frederick Sanger's sequencing method enabled precise DNA sequencing, which was crucial for the Human Genome Project. The 1980s saw next-generation sequencing, which reduced genome sequencing costs and time. Single-cell sequencing and CRISPR-Cas9 are also some important milestones that are driving genetic research.
- What is the impact of AI on clinical trials and antibody development?
AI has enabled the swift identification of promising drug candidates and the efficient development of new antibodies. This has led to faster initiation of clinical trials and significant advancements in therapeutic development.
- What are the ethical considerations with respect to using AI in genetic research?
Ethical concerns include data privacy, algorithmic bias, and its use in decision-making processes. Ensuring patient rights and transparency in AI-driven research is crucial to building trust and mitigating potential risks.